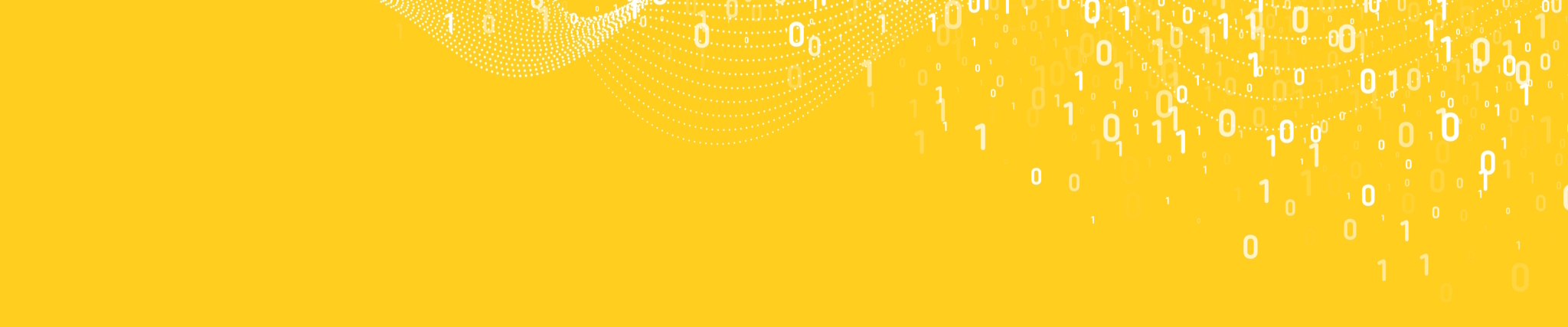
Technology
Beyond State-of-the-Art Technology
In the increasingly complex world of IT and industrial networks, we cannot rely solely on pre-set rules, baselines and tools to identify both known and, as yet, unknown threats. That’s why we invest considerable resources into our technology, innovation and research.
For us, artificial intelligence (AI) is not just an empty marketing buzzword, but is a powerful tool that provides our product with the extremely important ability to protect our users against new and highly sophisticated threats. Additionally, we do not think of machine learning as a self-serving operation, but as an essential ally for the teams that take care of the security and full functionality of the networks and infrastructures of our customers.
When it comes to research, we do not look at it merely as a formulaic element of our company’s presentation, but the only possible way forward in the ongoing fight against cyber threats. The best technology is created by the best people.
Overview
- - Visibility
- - Detection
- - Response
- - OT/SCADA/ICS
- - Research
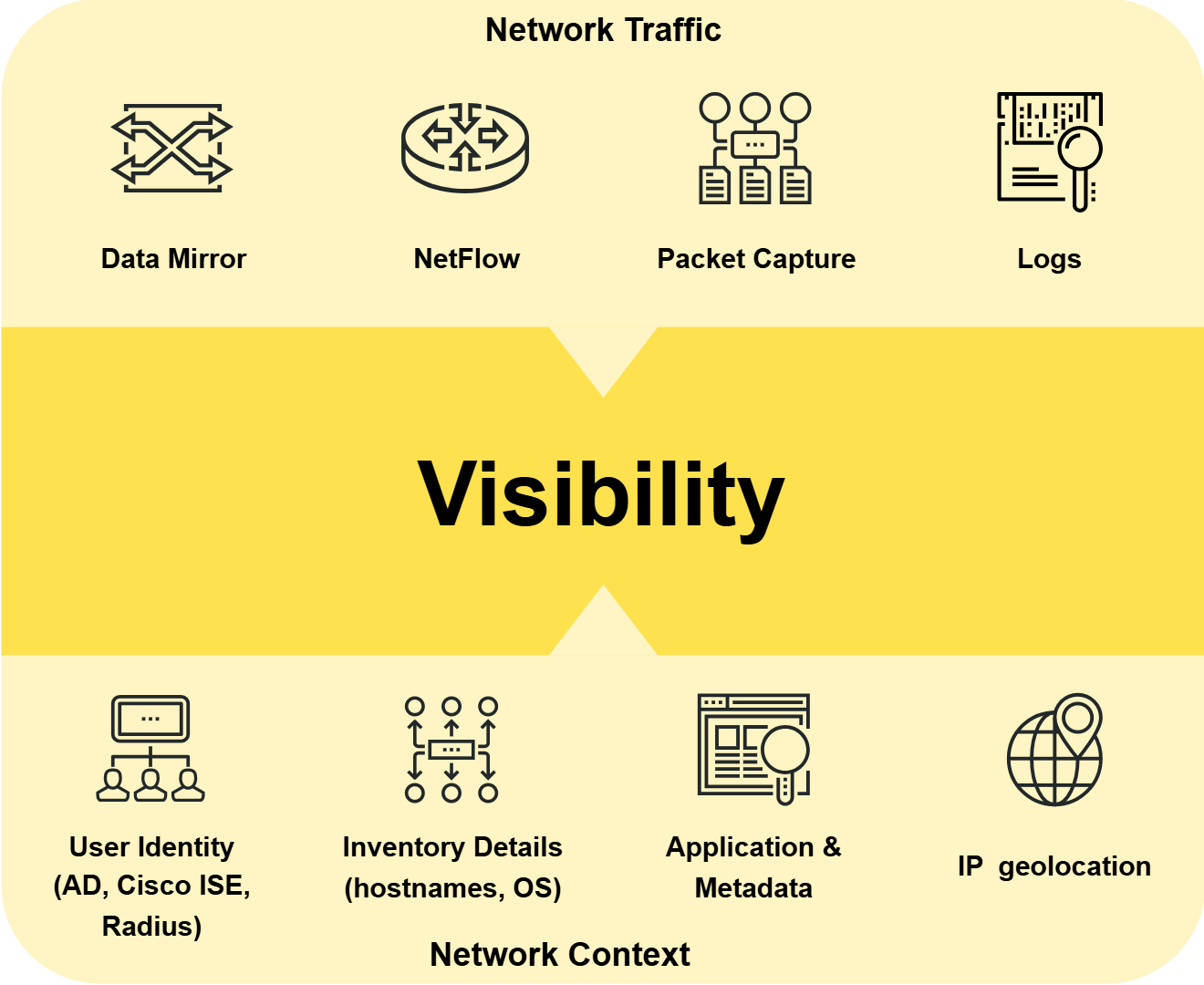
Visibility
GREYCORTEX Mendel provides you with the ability to visualize every device in your network in real time, so you can see exactly what devices are in the network – including with whom they are communicating, when, how much data they are sending and receiving, on what protocol, including its metadata, meaning that, thanks to our solution, security and operational events finally come with full and detailed context, user identities and any related threat intelligence.
But it doesn’t end there because Mendel takes this visualization a step further. With advanced filtering, combining more than 25 parameters, using logic operators, every device can not only be seen, but its communications on the network can also be thoroughly investigated, making root cause analysis, threat hunting, and network troubleshooting a breeze.
Detection
GREYCORTEX Mendel uses network detection and response to analyze and detect advanced, unknown attacks (APTs) infecting other devices, downloading Torrents, scanning for open ports, or communicating with the command and control server across your entire network in real-time. These actions create communications traffic within the network that is anomalous to “normal” network traffic.
Mendel consists of several cutting-edge technologies and detection engines:
- Intrusion Detection System (IDS)
- Deep Packet Inspection (DPI)
- Network Behavior Analysis (NBA) based on Artificial Intelligence
- Encrypted Traffic Analysis
- Network and Application Performance Monitoring (NPM, APM)
- Event Correlation (EC)
- Risk Assessment (RA)
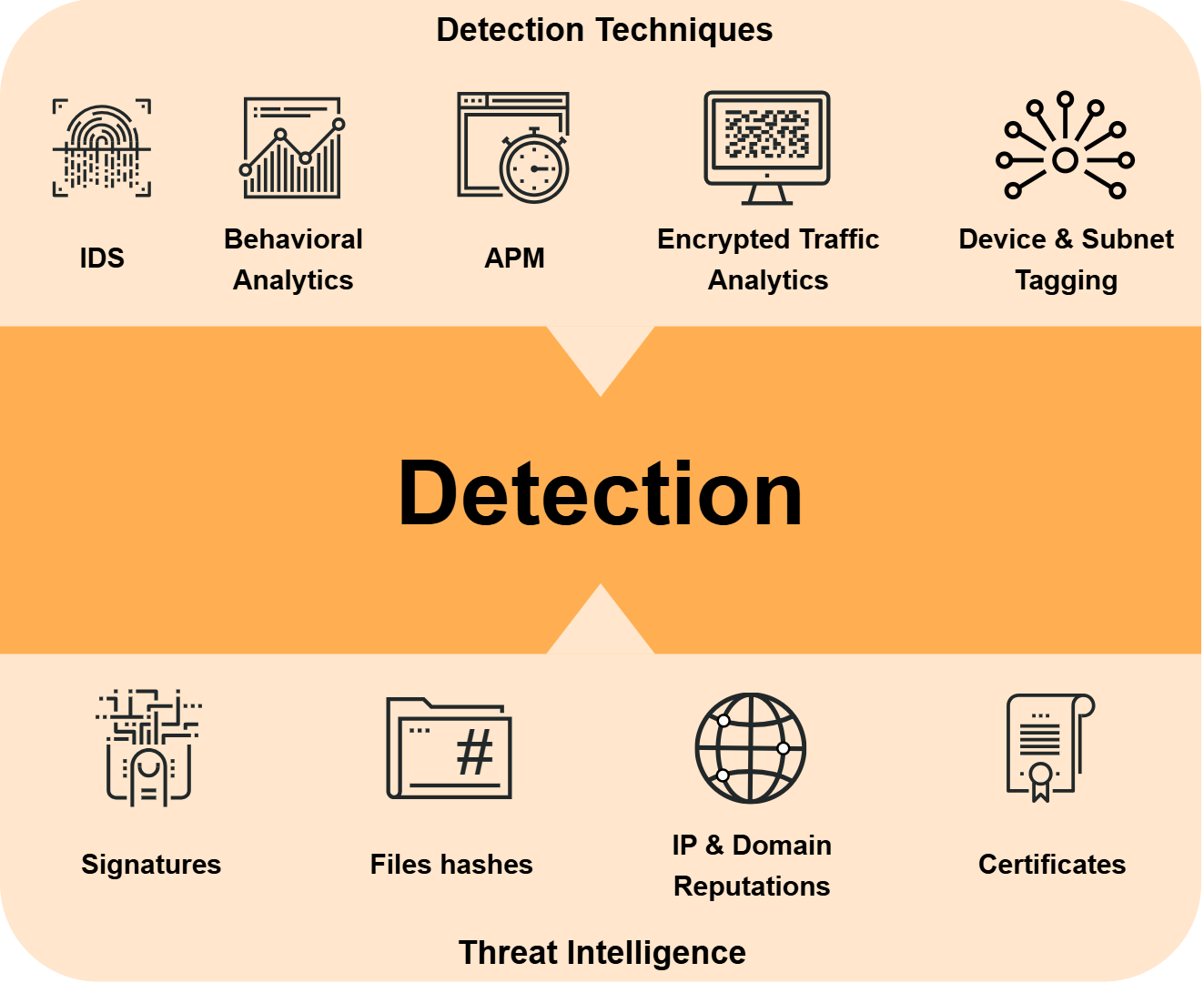
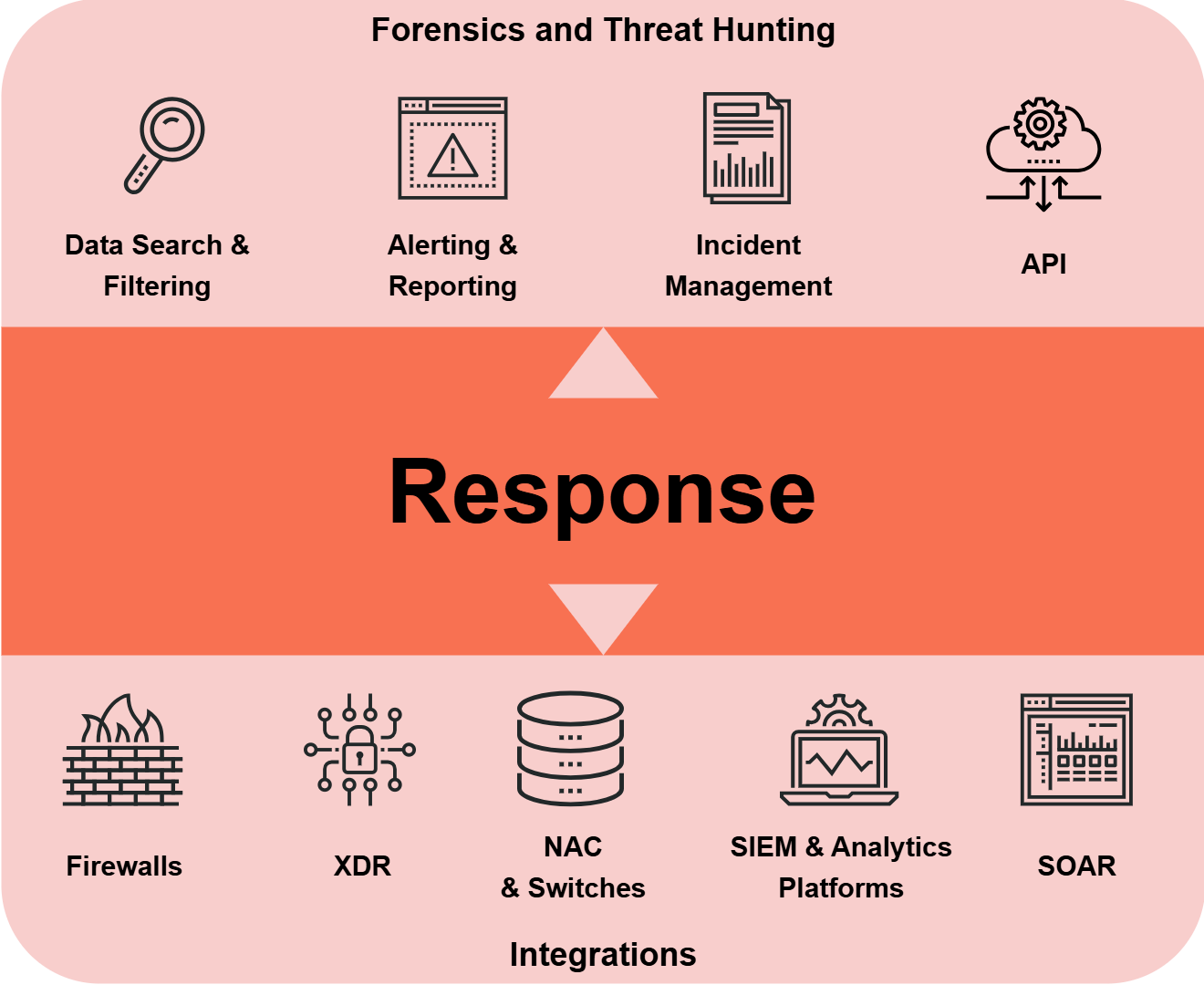
Response
Risk and correlation analysis combines several detected events together into a single incident and assesses the risk ratings of your network, subnets, hosts, and services. Incident management features allow several analysts to work on an issue at the same time, or balance the workload within the team.
For those working with SIEM systems, GREYCORTEX Mendel can export flow data and events to the SIEM for further investigation. Analysts can also return from the SIEM to GREYCORTEX Mendel using just one click for more details.
Mendel integrates with the security tools that are already in your network, like firewalls, access control systems, and other active security tools, so you can respond to attacks, conduct investigations, manage all incidents, and block any malicious communications from a single interface.
OT — SCADA/ICS
Our solution learns the patterns of your typical network behavior and adapts its model to the current hour of the day and week, detecting who is communicating with whom, when, and with what time frequency, also including the commands, data variables, and their values.
By creating behavior models for all physical and logical devices and equipment, including every station, service, and communication channel between devices in the OT network, Mendel can detect all anomalies. At the same time, it combines specific signatures for the detection of known threats and our detection signatures to identify approximately 300 types of industrial and critical infrastructure attacks on the most commonly used OT protocols.
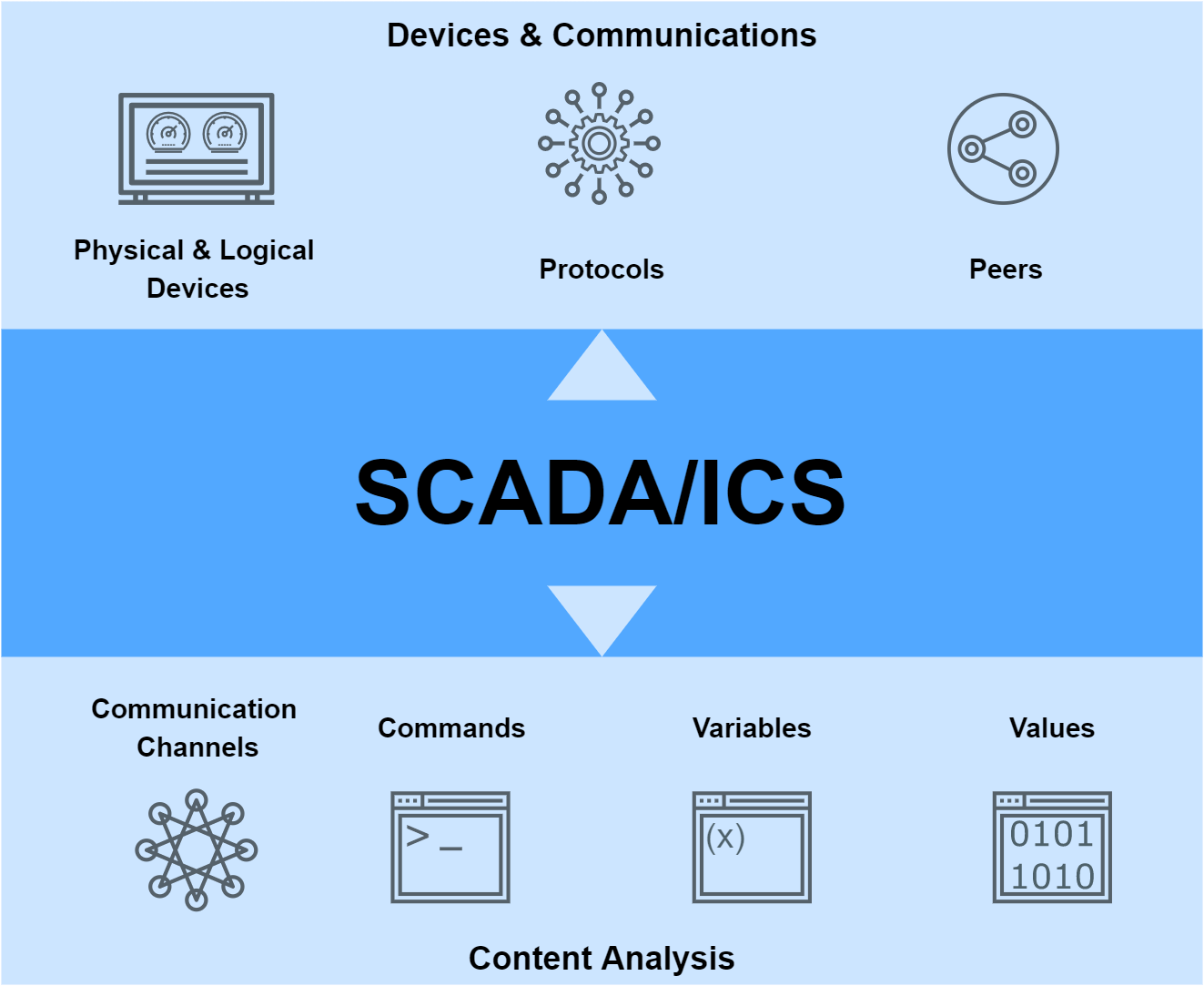
Research
GREYCORTEX Mendel is based on 10 years of extensive academic and industrial research, and designed using the same technology that has proved successful in multiple NIST Challenges. GREYCORTEX partners with leading research institutions worldwide in the fields of artificial intelligence, machine learning, and cybersecurity for both IT and industrial environments to ensure we provide the most up-to-date and powerful technology available on the market.
Our research interests lie in cybersecurity for IT and OT networks, such as SCADA, IoT, industrial, medical, transport, and wireless technologies that do not have end-point protection (antivirus), applying the techniques of machine learning and, especially, anomaly detection.